大家可以查看原文:https://mp.weixin.qq.com/s/2JYkT8a-HdJ2LePVJ3Vlag
关注我微信公众号
# Ordering
现实生活中时间可以记录事情发生的时刻、比较事情发生的先后顺序。
分布式系统的一些场景也需要记录和比较不同节点间事件发生的顺序。
如数据写入先后顺序,事件发生的先后顺序等等。
## 关系
复习下离散数学中关系:
假设A是一个集合 {1,2,3,4} ;R是集合A上的关系,例如{<1,1>,<2,2>,<3,3>,<4,4>,<1,2>,<1,4>,<2,4>,<3,4>}
- 自反性:任取一个A中的元素x,如果都有<x,x>在R中,那么R是自反的。
- <1,1>,<2,2>,<3,3>,<4,4>
- 对称性:任取一个A中的元素x,y,如果<x,y> 在关系R上,那么<y,x> 也在关系R上,那么R是对称的。
- 反对称性:任取一个A中的元素x,y(x!=y),如果<x,y> 在关系R上,那么<y,x> 不在关系R上,那么R是反对称的。
- 对于 <1,2>,有 <2,1> 不在R中;对于<2,4> 有<4,2>不在R中;对于<3,4> 有<4,3> 不在 R中,满足。
- 传递性:任取一个A中的元素x,y,z,如果<x,y>,<y,z> 在关系R上,那么 <x,z> 也在关系R上,那么R是对称的。
- <1,1><1,2>在R中,并且<1,2>在R中;<1,1><1,4>在R中,并且<1,4>在R中;<2,2><2,4>在R中,并且<2,4>在R中;<3,3><3,4>在R中,并且<3,4>在R中;等等其他,满足。
- 完全性(全关系):包含了自反性;对集合A中所有<x,y>,都有关系x到y或y到x;
- R中并没有<1, 3>,所以不满足完全性
## 偏序The Partial Ordering
集合内只有部分元素之间是可以比较的。
偏序关系的定义(R为A上的偏序关系):
设R是集合A上的一个二元关系,若R满足:
- 反对称性:对任意x,y∈A,若xRy,且yRx,则x=y;
- 传递性:对任意x, y,z∈A,若xRy,且yRz,则xRz
- 自反性:对任意x∈A,有xRx;
一个partitial ordering关系满足的条件是自反的,反对称的和可传递的,因此在partitial ordering中,可能有两个元素之间是不相关的。
## 全序The Total Ordering
集合内只有部分元素之间是可以比较的。
比如:比如复数集中并不是所有的数都可以比较大小,那么“大小”就是复数集的一个偏序关系。
全序关系的定义:
- 反对称性:对任意x,y∈A,若xRy,且yRx,则x=y;
- 传递性:对任意x, y,z∈A,若xRy,且yRz,则xRz
- 完全性(total relation全关系):对任意x,y∈A,由xRy或yRx (包括了自反性)
> 完全性本身也包括了自反性,所以全序关系是偏序关系。
> 所以偏序中满足完全性就是全序了。
一个total ordering关系满足的条件是反对称的,可传递的和完全性,因此在total ordering中,两个元素一定是有关系的,要么是a<>b或b<>a。
## happens before
在分布式系统中,一个进程包含一系列的事件,对于同一进程内的事件,如果a happens before b,那么a发生在b之前。并且,假定收或发消息都是一个事件。
happens before的定义如下(用->表示)
- 如果a和b在同一进程中,并且a发生在b之前,那么a->b
- 如果a是一个进程发消息的事件,b是另一个进程接收这条消息的事件,则a->b
- 如果a->b且b->c,那么a->c。
- 如果同时不满足a->b,且b->a,那么说a和b是并发的concurrent

[图来自Time, Clocks, and the Ordering of Events in a Distributed System]
以一个例子来说明happens before关系,如上图,垂直线上代表一个进程,从下往上,时间依次增加,水平的距离代表空间的隔离。原点代表一个事件,而曲线代表一条消息。
从图中很容易地看出,如果一个事件a,能通过进程的线和消息线,到达b,那么a->b。
在图中,p3和q4是并行的事件,因为,只有到了p4才能确定q4的发生,而q3也只能确定p1发生。
# clock时钟
## 物理时钟
### 晶振和时钟偏移
计算机有固定频率晶体的震荡次数,晶体的振荡周期决定了单机的时钟精度。
时钟频率也可能因为温度等外部因素导致时钟偏移,普通的石英晶体的漂移大约$10^{-6}$
原子钟的漂移约为 $10^{-13}$
所以原子钟精度远远高于石英晶体。
### 分布式下带来的问题
不同机器上的物理时钟难以同步,导致无法区分在分布式系统中多个节点的事件时序。即使设置了 NTP 时间同步节点间也存在毫秒级别的偏差,因而分布式系统需要有另外的方法记录事件顺序关系。
1978年Lamport在《Time, Clocks and the Ordering of Events in a Distributed System》中提出了逻辑时钟的概念,来解决分布式系统中区分事件发生的时序问题。
## 逻辑时钟Logical clocks
逻辑时钟指的是分布式系统中用于区分事件的发生顺序的时间机制。 从某种意义上讲,现实世界中的物理时间其实是逻辑时钟的特例。
Logical Clock解决的问题是找到一种方法,给分布式系统中所有时间定一个序,这个序能够正确地排列出具有因果关系的事件(注意,是不能保证并发事件的真实顺序的),使得分布式系统在逻辑上不会发生因果倒置的错误。因果一致性
### Lamport timestamps
#### 论文
[Time, Clocks, and the Ordering of Events in a Distributed System](https://amturing.acm.org/p558-lamport.pdf)
#### Lamport timestamps
Leslie Lamport 在1978年提出逻辑时钟的概念,并描述了一种逻辑时钟的表示方法,这个方法被称为Lamport时间戳(Lamport timestamps)。
分布式系统中按是否存在节点交互可分为三类事件:
- 发生在节点内部
- 发送事件
- 接收事件
时钟的定义如下
- 对于一个进程i,Ci(a)表示进程i中事件a的发生时间
- 对于整个系统来讲,对于任意的事件b,其发生时间为C(b),当b为进程j的事件时,则C(b) = Cj(b)
为了使得事件按照正确的排序,需要使得如果事件a发生在事件b之前,那么a发生的时间要小于b,如下
```
for any events a, b
if a->b then C(a) < C(b)
```
根据关系->的定义,我们可以得出
- 如果a和b都是进程i中的事件,且a发生在b之前,那么Ci(a) < Ci(b)
- 如果事件a发送消息给事件b,a属于进程i,b属于进程j,那么Ci(a) < Cj(b)

为了让系统满足上述条件,在实现中,需要满足以下原则
- 对于每个进程,相邻的事件的时钟要增加1
- (a) 如果事件a是进程i发送消息m的事件,发送时带时间戳Tm = Ci(a),(b)事件b是进程j接受消息m的事件,那么事件b的取值为max(进程b的当前时钟,Tm+1)
假设有事件a、b,C(a)、C(b)分别表示事件a、b对应的Lamport时间戳,如果a->b,则C(a) < C(b),a发生在b之前(happened before)。
所以Lamport timestamps原理如下:
- 每个事件对应一个Lamport时间戳,初始值为0
- 如果事件在节点内发生,时间戳加1
- 如果事件属于发送事件,时间戳加1并在消息中带上该时间戳
- 如果事件属于接收事件,时间戳 = Max(本地时间戳,消息中的时间戳) + 1
> 通过该定义,事件集中Lamport时间戳不等的事件可进行比较,我们获得事件的偏序关系(partial order)。

上图更形象的解释了事件之间的关系。
以B4事件为基准:
- B4左边深灰色的区域的事件,都发生在B4前,和B4具有因果关系,这些事件属于与B4因果关系中的因(cause)
- B4右边的深红色区域的事件,都发生在B4后,和B4具有因果关系,这些事件属于与B4因果关系中的果(effect)
- B4上下的白色区域是跟B4无关的事件,可以认为是并发关系(concurrent)
- 在浅灰色和浅红色区域中的事件,C2、A3两个事件与B4是并行关系,根据Lamport timestamps的定义,将他们判定为与B4具前后关系。(所以Lamport timestamps并不能严格的表示并行关系)
#### Lamport timestamps与偏序关系
Lamport timestamps只保证因果关系(偏序)的正确性,不保证绝对时序的正确性。
#### Lamport logical clock
由于Lamport timestamps只能得到偏序关系,如果要得到全序关系,就需要给Ci(a) = Cj(b)的事件定一个先后顺序。
total order的事件关系=>定义如下:
如果事件a发生在进程Pi,事件b发生在进程Pj,那么当满足下列两者条件之一时,a=>b
- Ci(a) < Cj(b)
- Ci(a) = Cj(b) 且 Pi < Pj
根据以上条件,对于任意的两个事件,都能判断出它们之间的关系,因此是total ordering的。
当Lamport timestamp一致时,通过义Pi < Pj来定义顺序,确保分布式场景下各个进程间发生的事件的全序定义。至于Pj < Pj:可采用不同的方式,Lamport Logical Clock提到的 arbitrary total ordering。
### vector clock
Lamport timestamp得到的是全序关系,但无法严格表示对于没有因果关系、存在同时发生关系(concurrent)的事件。
Vector clock是在Lamport timestamp基础上改进的一种逻辑时钟方法,它构不但记录本节点的Lamport timestamp,同时也记录了其他节点的Lamport timestamp。
原理如下:
- 本地vector clock的clock数组中每一个逻辑时间(clock)对应一个进程的clock
- 初始化vector clock中每一个逻辑时间为0;
- 每一次处理内完内部事件,将vector clock中自己的逻辑时间戳+1;
- 每发送一个消息的时候,将vector clock中自己的逻辑时间+1,且将其和消息一起发送出去
- 每接收到一个消息的时候,需要将本地的vector clock中自己的逻辑时间戳+1,且将自己vector clock中的逻辑时间和消息中携带的进行比较,取最大的更新本地vector clock中的逻辑时间。

[图来源于wikipedia](https://en.wikipedia.org/wiki/Vector_clock)
vector clock判定并发关系:
- 事件i、事件j对应的vector clock中,每一个进程Pk的逻辑时间戳都满足Vi[Pk]<Vj[Pk]时,我们称事件i happen before事件j;
- vector clock中,存在P1、P2,使得Vi[P1]<Vj[P1],Vi[P2]>Vj[P2],我们称事件i和事件j是并发关系(没有因果关系);
和之前lamport timestamp的一样,以B4事件为基准(vector clock为[A:2,B:4,C:1]),根据vector clock的判定,可以判断出
- 灰色区域的事件happens before B4事件,B4事件happens before红色区域的事件
- 白色区域与B4事件没有因果关系。
特性:
- vector clock不需要在节点之间同步时钟,不需要在所有节点上维护一段数据的版本数;
- 缺点是时钟值的大小随着节点增多和时间不断增长
### version vector
分布式系统多个副本被同时更新时,会导致副本之间数据的不一致。version vector用于来发现这些不一致的冲突。
version vector只能发现冲突,无法解决冲突;当然也可以通过再添加一个维度信息timestamp,发生冲突时进行比较,但是又回到了物理时钟不同步的问题。
下图展示了数据由不同副本处理后导致的不同版本冲突。
D5时发现了数据的冲突,这时会将不同版本数据都存储下来,一般由客户端来解决冲突。

**version vector与vector clock的差异**
- vector clocks 使用 receive和send 方法来更新clock,而version vector使用sync方法来更新。
- vector clocks是给事件定序的,确定事件的因果关系;而version vector是确定同一个数据不同版本的因果关系。
# 分布式与时钟
分布式系统中,每个节点的物理时钟是不同步的,都有一定的差异。
这样就带来了一些分布式系统实现的难题,如基于MVCC实现的事务,基于MVCC实现事务会要求版本之间能判断先后顺序,只有确定先后才知道应该用哪一个版本的数据,确定先后顺序就涉及到时间,而不同机器之间的本地时钟是无法保证一致的,所以这就需要确保时钟的同步。
而通常解决方案有两种:
- 中心化的时钟方案,如Timestamp oracle(TSO)
- 无中心化的时钟方案,如google True Time,Hybrid Logic Time
## Timestamp oracle
如果我们整个系统不复杂,而且没有跨全球的需求,这时用一台中心授时服务就可以了。
如TiDB使用的就是TSO方案,tipb作为一个TSO集群,来提供授时服务。
使用TSO的好处在于因为只有一个中心授时,所以我们一定能确定所有时间的时间,但TSO需要关注几个问题:
- 网络延时:因为所有的事件都需要从TSO获取时间,所以TSO只适合小集群部署,不能是那种全球级别的数据库
- 性能:每个事件都需要从TSO获取时间,所以TSO需要非常高的性能
- 容错:TSO是一个单点,需要考虑节点的failover
## True Time
由于节点间NTP是有偏差的,且可能出现时间回退的情况,所以NTP无法准确的判定事件的全序关系。在Google Spanner里面,通过引入True Time来解决了分布式时间问题。
### True Time实现
Spanner通过使用GPS + 原子钟atomic clock来对集群的机器时间进行校对,保证了集群机器的时间戳差距不会超过一个上限值(ε)。
用两种技术来处理,是因为导致这两种技术的失败的原因是不同的。
- GPS会有一个天线,电波干扰会导致其失灵。原子钟很稳定。
- 当GPS失灵的时候,原子钟仍然能保证在相当长的时间内,不会出现偏差。
### API
- TT.now() : 返回一个当前时间,其位于范围区间[earliest,latest]
- TT.after(t) : 当前时间是否在t之后
- TT.before(t) : 当前时间是否在t之前
虽然spanner引入了TrueTime可以得到全球范围的时序一致性,但由于TrueTime返回的时间仍然有一定的偏差,如果要给两个事件定序,就需要等待2个偏差的时间间隔,来确保其先后顺序。
- 事件a:[Tai, Taj], Taj-Tai=ε
- 事件b:[Tbi, Tbj], Tbj-Tbi=ε
- 所以要确定b>a, 那么就要确保Tbi > Taj, 就需要在事件b进行等待,以确保:`事件b时间 - 事件a时间 > 2ε`
## Hybrid logical clock
### HLC
[Logical Physical Clocks and Consistent Snapshots in Globally Distributed Databases](https://cse.buffalo.edu/tech-reports/2014-04.pdf)
TrueTime 需要硬件的支持,所以有一定的成本,而HLC无需硬件支持也能解决分布式下时间问题。
HLC同时使用了物理时钟和逻辑时钟(physical clock + logical clock),能够保证单点的时间发生器是单调递增的,同时能够尽量控制不同节点之间的时钟偏差在规定的偏差范围内。
判断两个事件的先后顺序:先判断物理时间,再判断逻辑时间。
#### HLC的算法
l.j维护的是节点j当前已知的最大的物理时间(wall time),c.j则是当前的逻辑时间。
```
// 在节点j上面:初始化: l.j = 0,c.j = 0。
initially l.j :=0; c.j := 0
// 本地事件或者发送消息时,
// 如果本地时钟pt大于当前的混合逻辑时钟的l,
// 则将l更新成本地时钟,将c清零。
// 否则,l保持不变,将c加1。
Send or local event
{
l'.j := l.j;
l.j := max(l'.j, pt.j); // 本地物理时间pt
if (l.j = l'.j) then
c.j := c.j+1
else
c.j := 0;
Timestamp with l.j, c.j
}
// 收到消息时
// l在 当前的逻辑时钟的l、机器的本地时钟pt、收到消息里面带的l,三者中取最大的。
// 如果l部分是更新为本地时钟了,则将c清零。否则,c取较大的那个l对应到的c加1。
Receive event of message m
{
l'.j := l.j;
l.j := max(l'.j, l.m, pt.j);
if (l.j = l'.j = l.m) then
c.j := max(c.j, c.m) + 1
elseif (l.j=l'.j) then
c.j := c.j + 1
elseif (l.j=l.m) then
c.j := c.m + 1
else
c.j := 0
Timestamp with l.j, c.j
}
```
#### 特性
HLC算法保证了HLC时间有如下特性:
- 事件e发生在事件f之前,那么事件e的HLC时间一定小于事件f的HLC时间:(l.e, c.e) < (l.f, c.f)
- 本地WallTime大于等于本地物理时间(l.e ≥ pt.e):HLC时间总是不断递增,不会随着物理时间发生回退。
- 对事件e,l.e是事件e能感知的到的最大物理时间值:如果l.e > pt.e,那么一定存在着一个发生在e之前的事件g,有pt.g=l.e。简单来说是如果出现l.e > pt.e肯定是因为有一个HLC时间更大的的节点把当前节点的HLC时间往后推了。
- WallTime和物理时钟的偏差是有界的(ε ≥ |pt.e - l.e| ):因为节点之间通过NTP服务校时,那么节点之间的物理时钟偏差一定小于某个值ε。那么对于任一事件b和e,如果b hb e,那么事件b的物理时间pt.b一定满足pt.e + ε ≥ pt.b。结合特性3存在一个事件g满足,l.e = pt.g。那么 pt.e + ε ≥ l.e=pt.g > pt.e。
### 开源实现
CockroachDB采用基于NTP时钟同步的HLC去中心化方案。
#### 时钟同步
所有节点间的RPC消息都会把时间戳带入到消息中,接收到消息的节点会通过消息中的时间戳更新自己的时间, 从而达到节点间时间同步的效果。
#### 代码分析
参考:https://github.com/cockroachdb/cockroach/blob/v1.1.3/pkg/util/hlc/hlc.go
**HLC定义**
```
// Timestamp represents a state of the hybrid logical clock.
type Timestamp struct {
// Holds a wall time, typically a unix epoch time
// expressed in nanoseconds.
WallTime int64 `protobuf:"varint,1,opt,name=wall_time,json=wallTime" json:"wall_time"`
// The logical component captures causality for events whose wall
// times are equal. It is effectively bounded by (maximum clock
// skew)/(minimal ns between events) and nearly impossible to
// overflow.
Logical int32 `protobuf:"varint,2,opt,name=logical" json:"logical"`
}
```
- WallTime:本地已知物理时钟
- Logical:逻辑时钟
- Timestamp:HLC,单调递增
**获取物理时钟**
```
// PhysicalNow returns the local wall time. It corresponds to the physicalClock
// provided at instantiation. For a timestamp value, use Now() instead.
func (c *Clock) PhysicalNow() int64 {
c.mu.Lock()
defer c.mu.Unlock()
return c.getPhysicalClockLocked()
}
// getPhysicalClockLocked returns the current physical clock and checks for
// time jumps.
func (c *Clock) getPhysicalClockLocked() int64 {
// physicalClock 就是 UnixNano
newTime := c.physicalClock()
if c.mu.lastPhysicalTime != 0 {
interval := c.mu.lastPhysicalTime - newTime
// 检查时钟是否回退
if interval > int64(c.maxOffset/10) {
c.mu.monotonicityErrorsCount++
log.Warningf(context.TODO(), "backward time jump detected (%f seconds)", float64(-interval)/1e9)
}
}
c.mu.lastPhysicalTime = newTime
return newTime
}
// UnixNano returns the local machine's physical nanosecond
// unix epoch timestamp as a convenience to create a HLC via
// c := hlc.NewClock(hlc.UnixNano, ...).
func UnixNano() int64 {
return timeutil.Now().UnixNano()
}
```
**获取当前HLC时钟**
```
// Now returns a timestamp associated with an event from
// the local machine that may be sent to other members
// of the distributed network. This is the counterpart
// of Update, which is passed a timestamp received from
// another member of the distributed network.
func (c *Clock) Now() Timestamp {
c.mu.Lock()
defer c.mu.Unlock()
if physicalClock := c.getPhysicalClockLocked(); c.mu.timestamp.WallTime >= physicalClock {
// The wall time is ahead, so the logical clock ticks.
c.mu.timestamp.Logical++
} else {
// Use the physical clock, and reset the logical one.
c.mu.timestamp.WallTime = physicalClock
c.mu.timestamp.Logical = 0
}
return c.mu.timestamp
}
```
- 如果当前物理时钟小于WallTime,则将逻辑时钟+1
- 如果当前物理时钟大于WallTime,则更新WallTime为当前物理时钟,且将逻辑时钟设置为0
**节点时钟同步**
节点之间通过在RPC请求中携带HLC时间来进行时钟同步。
```
// sendSingleRange gathers and rearranges the replicas, and makes an RPC call.
func (ds *DistSender) sendSingleRange(
ctx context.Context, ba roachpb.BatchRequest, desc *roachpb.RangeDescriptor,
) (*roachpb.BatchResponse, *roachpb.Error) {
......
br, err := ds.sendRPC(ctx, desc.RangeID, replicas, ba)
if err != nil {
log.ErrEvent(ctx, err.Error())
return nil, roachpb.NewError(err)
}
// If the reply contains a timestamp, update the local HLC with it.
if br.Error != nil && br.Error.Now != (hlc.Timestamp{}) {
ds.clock.Update(br.Error.Now)
} else if br.Now != (hlc.Timestamp{}) {
ds.clock.Update(br.Now)
}
......
}
// Update takes a hybrid timestamp, usually originating from
// an event received from another member of a distributed
// system. The clock is updated and the hybrid timestamp
// associated to the receipt of the event returned.
// An error may only occur if offset checking is active and
// the remote timestamp was rejected due to clock offset,
// in which case the timestamp of the clock will not have been
// altered.
// To timestamp events of local origin, use Now instead.
func (c *Clock) Update(rt Timestamp) Timestamp {
c.mu.Lock()
defer c.mu.Unlock()
// 如果本地物理时间pt
physicalClock := c.getPhysicalClockLocked()
// 大于本地WallTime且大于rt.WallTime:
// 更新本地WallTime=pt,且logical=0
if physicalClock > c.mu.timestamp.WallTime && physicalClock > rt.WallTime {
// Our physical clock is ahead of both wall times. It is used
// as the new wall time and the logical clock is reset.
c.mu.timestamp.WallTime = physicalClock
c.mu.timestamp.Logical = 0
return c.mu.timestamp
}
// In the remaining cases, our physical clock plays no role
// as it is behind the local or remote wall times. Instead,
// the logical clock comes into play.
// 如果rt.WallTime > 本地WallTime:
// 检查rt.WallTime与pt是否大于时钟偏差;
// 本地WallTime=rt.WallTime,logical++
if rt.WallTime > c.mu.timestamp.WallTime {
offset := time.Duration(rt.WallTime-physicalClock) * time.Nanosecond
if c.maxOffset > 0 && offset > c.maxOffset {
log.Warningf(context.TODO(), "remote wall time is too far ahead (%s) to be trustworthy - updating anyway", offset)
}
// The remote clock is ahead of ours, and we update
// our own logical clock with theirs.
c.mu.timestamp.WallTime = rt.WallTime
c.mu.timestamp.Logical = rt.Logical + 1
} else if c.mu.timestamp.WallTime > rt.WallTime {
// 如果本地WallTime>rt.WallTime:logical++
// Our wall time is larger, so it remains but we tick
// the logical clock.
c.mu.timestamp.Logical++
} else {
// Both wall times are equal, and the larger logical
// clock is used for the update.
if rt.Logical > c.mu.timestamp.Logical {
c.mu.timestamp.Logical = rt.Logical
}
c.mu.timestamp.Logical++
}
return c.mu.timestamp
}
```
# 参考
- [Lamport timestamp](https://en.wikipedia.org/wiki/Lamport_timestamp)
- [Time, Clocks, and the Ordering of Events in a Distributed System](https://amturing.acm.org/p558-lamport.pdf)
- [Vector clock](https://en.wikipedia.org/wiki/Vector_clock)
- [Version vector](https://en.wikipedia.org/wiki/Version_vector)
- [Why Logical Clocks are Easy](https://queue.acm.org/detail.cfm?id=2917756)
- [Version Vectors are not Vector Clocks](https://haslab.wordpress.com/2011/07/08/version-vectors-are-not-vector-clocks/)
- [Vector Clocks Revisited](https://riak.com/posts/technical/vector-clocks-revisited/)
有疑问加站长微信联系(非本文作者))
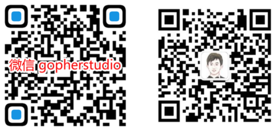